House Prices: Advanced Regression Techniques
Predict sales prices and practice feature engineering, RFs, and gradient boosting
www.kaggle.com
타이타닉 대회를 마무리하고, 다음으로 도전해볼 만한 캐글 컴퍼티션이 뭐가 있을까 찾아보다가 이 집값을 예측하는 대회를 찾았다. 오호, 집값을 예측한다고? 나도 그럼 부동산으로 돈 한번 땡겨 볼 수 있을까? 하는 생각에 신나게 대회 참가 버튼을 눌렀다.
타이타닉보다는 훨씬 데이터도 많고, (하지만 여전히 적음) 다루기도 어렵다. 그리고 타이타닉과 같은 'Classification', 분류 문제가 아니라 'Regression' 회귀 문제이다. Regression 문제는 정답이 어떤 실수 형태로 주어져서, 이 값을 예측하는 문제이다. 반면 Classification 문제는 타이타닉처럼 데이터가 어떤 종류인가 (생존인가, 사망인가) 를 맞추는 문제이다. 그럼 생존 확률을 맞추라그러는거는 뭐냐? 여기서 LogisticRegression 을 처음 들어본 사람은 멘붕이 온다. LogisticRegression은 이름에는 Regression 이라고 되어 있지만, 사실은 Classification 문제에 사용한다. 쉽게 생각하면, 이 LogisticRegression은 해당 클래스의 확률 값을 찾는 Regression이어서 이름에는 Regression 이 들어가지만, Classification 문제에 사용된다고 생각하면 된다. Classification 이어도, 문제에 따라서는 확률 값을 제출하기를 요구하는 문제들도 있다.
1. 데이터 로드 (Load Data & Packages)
import pandas as pd
import numpy as np
from scipy.stats import norm
%matplotlib inline
import matplotlib.pyplot as plt
import seaborn as sns
import warnings; warnings.simplefilter('ignore')
패키지들을 로드해주고
train=pd.read_csv('train.csv', index_col='Id')
test=pd.read_csv('test.csv', index_col='Id')
submission=pd.read_csv('sammple_submission.csv', index_col='Id')
data=train
print(train.shape, test.shape, submission.shape)
마찬가지로 데이터를 불러와준다. 주피터 노트북 파일과 저 csv 파일들이 같은 폴더 안에 있을때 잘 작동한다. 여기서는 data 라는 변수에 train을 다시 저장했는데, 데이터를 보면 변수가 매우 많고, 손을 많이 댈 예정이라서 꼬일 수도 있기 때문에 data 라는 변수를 새로 만들었다.
print 문의 결과를 보자면
(1460, 80) (1459, 79) (1459, 1) 이라고 출력될 것이다.
총 1460 행의 train 데이터를 가지고 1459 행의 test 데이터의 집값을 예측해야 한다. 이때 변수는 79개... 로 꽤나 많이 주어진다. 손이 많이 갈 것이다.
2. 데이터 분석하기
2.1. 타겟 변수 확인 (Distribution of Target)
이번 대회는 Regression 문제인 만큼 더더욱 타겟 변수의 분포를 확인해야 할 필요성이 생겼다. 코드를 보면,
figure, (ax1, ax2) = plt.subplots(nrows=1, ncols=2)
figure.set_size_inches(14,6)
sns.distplot(data['SalePrice'], fit=norm, ax=ax1)
sns.distplot(np.log(data['SalePrice']+1), fit=norm, ax=ax2)
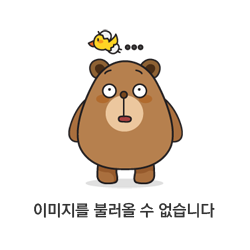
왼쪽 그래프를 대충 보아도 약 400000 정도 값이 중심인 것을 알 수 있다. 하지만 많은 데이터가 400000보다 왼쪽으로 치우쳐 있는데, 이렇게 데이터의 분포가 비대칭인 정도를 'Skewness' 라고 한다. 한국어로 번역하면 '왜도' 라고 한다. 이 Skewness의 꼬리가 오른쪽으로 길게 있으면 'Right Skewed' 또는 'Positive Skewed', 왼쪽으로 길게 있으면 'Left Skewed' 또는 'Negative Skewed' 라고 한다. 오른쪽이든 왼쪽이든 심한 비대칭은 머신러닝 알고리즘이 학습을 잘 하지 못하도록 방해하는 요소 중 하나이다. 대부분의 데이터가 왼쪽에 있다면, 오른쪽 꼬리 부분은 데이터가 적어서 학습이 잘 안될 가능성이 있다. 따라서 예측 결과물도 의심할 수 밖에 없다. 이런 상황에서 'Right Skewed' 를 해결하는 대표적인 방법이 바로 해당 변수에 로그를 취하는 것이다. 키야~ 로그에 취한다~
확실히 로그를 취하면 비대칭도가 줄어들고, 정규분포에 가깝게 데이터가 분포되어 있는 것을 확인할 수 있다. 우리는 이렇게, 머신러닝에게 로그를 취한 값을 타겟 변수로 주어서 예측하게끔 한 다음에, 마지막에 제출할 때만 지수 계산을 해서 제출하면 그만이다.
그럼 조금 이따가 타겟 변수에 로그를 취하고, 다른 그래프도 띄워보자.
2.2. 변수간 상관관계 확인 (Feature Correlation)
corr=data.corr()
top_corr=data[corr.nlargest(40,'SalePrice')['SalePrice'].index].corr()
figure, ax1 = plt.subplots(nrows=1, ncols=1)
figure.set_size_inches(20,15)
sns.heatmap(top_corr, annot=True, ax=ax1)
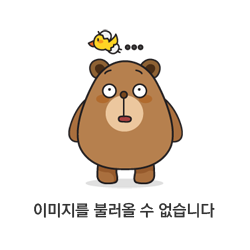
어떤 두 개의 변수 A와 B가 있을때, 변수 A 의 값이 커질때, 변수 B의 값도 커지면 이 둘은 양의 상관관계를 갖고 있다고 한다. 반대로, 변수 A의 값이 커질때, 변수 B의 값이 작아지면 이 둘은 음의 상관관계를 갖고 있다고 말한다. 그렇다면 상관관계가 0인 경우는? 그렇다. 변수 A가 어떻게 움직이든 변수 B에 별 영향이 없는 경우를 말한다. 이 상관관계를 측정하는 값을 '상관계수' 라고 말하고, 우리는 주로 '피어슨 상관계수' 를 이용해 상관계수를 분석한다. 이 그래프는 타겟 변수인 'SalePrice' 와 가장 큰 상관관계를 가진 40개의 변수를 표시하는 그래프이다. 그래프를 뜯어보면, 변수 'OverallQual' 이라는 변수가 상관계수 0.79로 타겟변수와 가장 큰 상관관계를 가지고 있는 것으로 나타났다. 전반적으로 OverallQual 이 증가하면, 집값도 증가한다고 볼 수 있다는 뜻이다.
sns.regplot(data['GrLivArea'], data['SalePrice'])
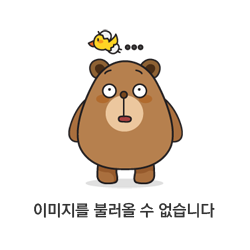
두번째로 큰 상관계수를 가진 'GrLivArea' 의 그래프를 띄워보면 위와 같다. 전체적으로 'GrLivArea' 가 증가하면 집값도 증가한다는 것을 확인할 수 있다. 다만 눈에 약간 거슬리는 부분이 있는데, 오른쪽 아래에 점 두개이다. 저 점들의 분포는 그래프 상의 저 직선으로 표현이 가능한데, 오른쪽 아래에 있는 저 두 점들은 전혀 다른 결과를 보여주고 있다. 저런 데이터들을 '이상치' (Outlier)로 간주하고 삭제해주는 것도 머신러닝의 정확도를 높일 수 있는 방법 중 하나이다.
train=train.drop(train[(train['GrLivArea']>4000) & (train['SalePrice']<300000)].index)
이제 본격적으로 데이터를 처리할 준비를 한다. 타이타닉에서 했던 것처럼 train 과 test 에 코드를 두번 쓰지 않고, train 과 test 를 묶어서 all_data 라는 변수에 저장한 후 이를 처리하고, 머신러닝에 학습시키기 전에 다시 자를것이다.
Ytrain=train['SalePrice']
train=train[list(test)]
all_data=pd.concat((train, test), axis=0)
print(all_data.shape)
Ytrain=np.log(Ytrain+1)
타겟 변수를 미리 Ytrain으로 빼서 저장하고 위에서 말한듯이 로그를 씌웠다. 로그를 씌우는데 +1 을 해주는 이유는 log0 의 값이 없기 때문이다. 공짜 집은 없겠지만 만약 Ytrain의 값중 하나가 0이라면..? log0은 마이너스 무한대로 커지기 때문에 +1씩 해주어서 이를 방지한다. 이를 'log1p' 라고 하기도 한다.
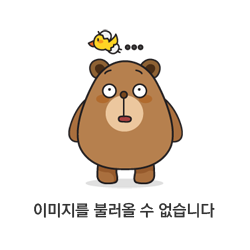
2.3. 전체 데이터에서 결측치 확인 (Check Missing Values)
이 데이터는 사실 상당히 깔끔하지만, 막상 꺼내어 보면 결측치가 참 많이 있다. 이는 캐글에서 데이터를 다운로드 받을 때 같이 딸려오는 'data description.txt' 파일을 읽어보면, 집에 해당 시설물이 없는 경우는 결측치로 처리되어 있음을 알 수 있다. 물론 중간에는 진짜 결측지도 있겠지만, 없는 경우가 더 많다. 따라서 결측치를 처리하는 방법이 매우 간단하다.
cols=list(all_data)
for col in list(all_data):
if (all_data[col].isnull().sum())==0:
cols.remove(col)
else:
pass
print(len(cols))
list(all_data) 를 사용하면 all_data 라는 데이터프레임의 열 이름을 리스트로 만들 수 있다. 다음 코드는 반복문을 통해서 all_data 에서 해당 열에 결측치가 없으면 리스트에서 그 열의 이름을 지운다. 그러면 남은 리스트에는 결측치가 있는 변수 이름만 남아있을 것이다. print 문으로 출력해보면 아래 코드에 있는 이름들이 나올 것이다.
for col in ('PoolQC', 'MiscFeature', 'Alley', 'Fence', 'FireplaceQu', 'GarageType', 'GarageFinish', 'GarageQual', 'GarageCond', 'BsmtQual', 'BsmtCond', 'BsmtExposure', 'BsmtFinType1', 'BsmtFinType2', 'MasVnrType', 'MSSubClass'):
all_data[col] = all_data[col].fillna('None')
for col in ('GarageYrBlt', 'GarageArea', 'GarageCars', 'BsmtFinSF1', 'BsmtFinSF2', 'BsmtUnfSF','TotalBsmtSF', 'BsmtFullBath', 'BsmtHalfBath', 'MasVnrArea','LotFrontage'):
all_data[col] = all_data[col].fillna(0)
for col in ('MSZoning', 'Electrical', 'KitchenQual', 'Exterior1st', 'Exterior2nd', 'SaleType', 'Functional', 'Utilities'):
all_data[col] = all_data[col].fillna(all_data[col].mode()[0])
print(f"Total count of missing values in all_data : {all_data.isnull().sum().sum()}")
첫번째, 집에 해당 시설물이 없는 경우 (범주형 변수), 이때는 결측치를 'None' 이라는 문자열로 채운다. (이 작은 따옴표 없는 None 과 문자열 'None' 은 다르다!)
두번째, 집에 해당 시설물이 없는 경우(수치형 변수), 이때는 결측치를 0으로 채운다. 차고면적=0 이면 차고 없음 이런식으로 생각할 수 있다.
세번째, 해당 시설물이 없다고 보기 힘든 경우에 있는 결측치, 이때는 결측치를 해당 열의 최빈값으로 채운다. 집에 외벽 시설이 없을리는 없고, 집이 판매가 되었는데 거래 타입이 정해지지 않을리는 없다.
2.4. 본격적으로 데이터 분석 (EDA)
데이터의 변수 이름을 유심히 뜯어보던 나는 몇 개의 새로운 변수를 만들기로 했다.
1) 총 가용면적 (Total SF Available)
figure, ((ax1, ax2), (ax3, ax4)) = plt.subplots(nrows=2, ncols=2)
figure.set_size_inches(14,10)
sns.regplot(data['TotalBsmtSF'], data['SalePrice'], ax=ax1)
sns.regplot(data['1stFlrSF'], data['SalePrice'], ax=ax2)
sns.regplot(data['2ndFlrSF'], data['SalePrice'], ax=ax3)
sns.regplot(data['TotalBsmtSF'] + data['1stFlrSF'] + data['2ndFlrSF'], data['SalePrice'], ax=ax4)
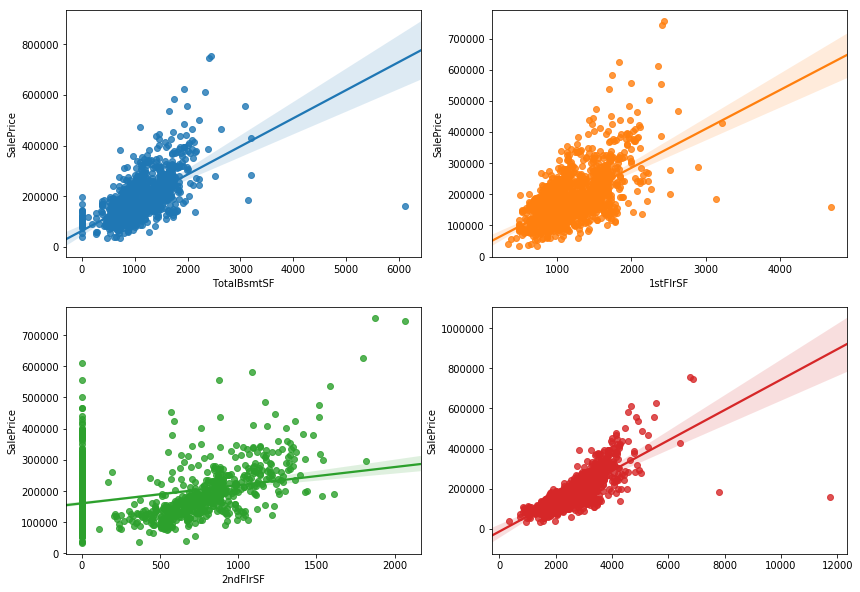
지하실, 1층, 2층 면적을 모두 합한 '총 면적' 이란 변수를 추가로 만들었다. 오른쪽 아래에 있는 그래프가 나머지 3개를 합한 면적을 나타낸 그래프인데, 상당히 타겟변수를 잘 설명할 수 있다. 상관관계도 꽤 높아보인다. 이는 총 면적이 증가하면, 집값이 더 비싸진다고 볼 수 있다. 이들을 더해서 'TotalSF' 라는 이름으로 저장해야겠다.
all_data['TotalSF']=all_data['TotalBsmtSF'] + all_data['1stFlrSF'] + all_data['2ndFlrSF']
all_data['No2ndFlr']=(all_data['2ndFlrSF']==0)
all_data['NoBsmt']=(all_data['TotalBsmtSF']==0)
추가로 'No2ndFlr' 과 'NoBsmt' 라는 변수를 만들어서 2층 없음, 지하실 없음 여부를 나타낼 수 있도록 했다.
2층면적을 나타내는 초록 그래프를 보면, 나타나있는 직선이 점들과 별로 맞지 않는 것처럼 보인다. 나는 그 이유를 아마 저 그래프의 0들 때문이라고 생각해서, 이 0들을 따로 분리해낸다면 상당히 예쁜 직선을 그릴 수 있을 것이라고 판단했다.
2) 총 욕실 수 (Bath)
figure, ((ax1, ax2), (ax3, ax4)) = plt.subplots(nrows=2, ncols=2)
figure.set_size_inches(14,10)
sns.barplot(data['BsmtFullBath'], data['SalePrice'], ax=ax1)
sns.barplot(data['FullBath'], data['SalePrice'], ax=ax2)
sns.barplot(data['BsmtHalfBath'], data['SalePrice'], ax=ax3)
sns.barplot(data['HalfBath'], data['SalePrice'], ax=ax4)
figure, (ax5) = plt.subplots(nrows=1, ncols=1)
figure.set_size_inches(14,6)
sns.barplot(data['BsmtFullBath'] + data['FullBath'] + (data['BsmtHalfBath']/2) + (data['HalfBath']/2), data['SalePrice'], ax=ax5)
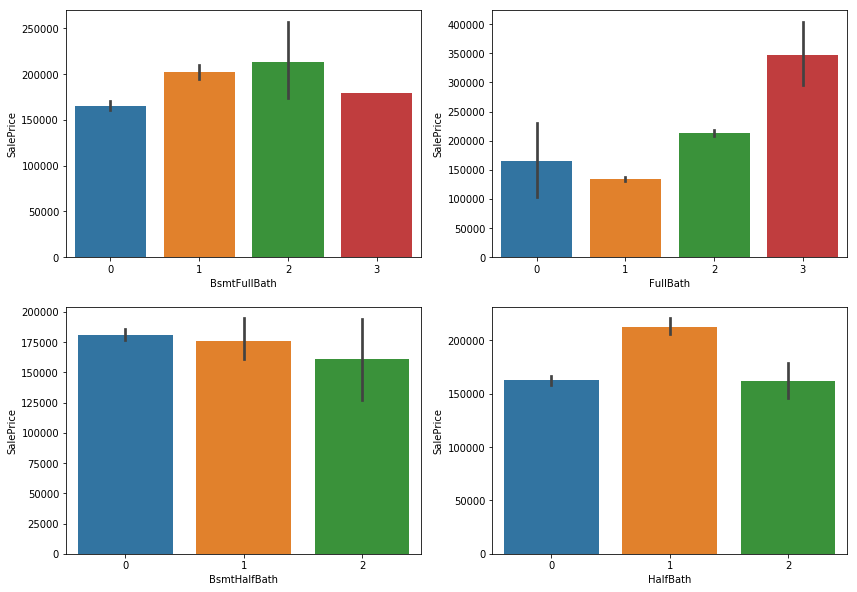
욕실 갯수는 'FullBath' 와 'HalfBath', 그리고 지하에 있는지 여부, 총 4개 열로 이루어져있었는데, 이들을 모두 더해 하나로 만들어 보았다. FullBath 는 욕조 및 샤워 시설이 포함되어있는 욕실이고, HalfBath는 변기와 세면대 정도 있는 간단한 욕실을 말한다. 이름에 맞게 FullBath는 1개로 카운트하고, HalfBath 는 0.5개로 카운트해서 모두 더했더니 다음과 같은 그래프를 얻을 수 있었다.

이를 보면 욕실 수가 많을수록 더 집값이 비싸진다고 볼 수 있다. (더 큰 집일수록 욕실수가 많을 것이라 생각한다). 하지만 여기서 특이한 점이 하나 있는데, 욕실 갯수가 5개, 6개인 집들은 막대그래프 위의 검정 세로선이 보이지 않는다. 에 이게뭐지? 할 수도 있지만, 저 검정 선은 편차를 의미한다. 그렇다면 편차가 없다는것이 무슨뜻이지? 값이 다른 데이터가 두개만 있어도 편차가 발생할텐데? 화장실 갯수가 5개, 6개인 집은 각각 하나씩밖에 없다는 뜻으로 볼 수 있다. 이들은 역시 outlier 로 판단하고 지워도 상관이 없다.
all_data['TotalBath']=all_data['BsmtFullBath'] + all_data['FullBath'] + (all_data['BsmtHalfBath']/2) + (all_data['HalfBath']/2)
3) 건축연도 + 리모델링 연도 (Year Built and Remodeled)
이 둘은 상당히 비슷한 분포로 데이터가 이루어져 있다. 하지만 나는 이 둘을 모두 포함하는 데이터를 하나 추가하고 싶었다.
figure, (ax1, ax2, ax3) = plt.subplots(nrows=1, ncols=3)
figure.set_size_inches(18,8)
sns.regplot(data['YearBuilt'], data['SalePrice'], ax=ax1)
sns.regplot(data['YearRemodAdd'], data['SalePrice'], ax=ax2)
sns.regplot((data['YearBuilt']+data['YearRemodAdd'])/2, data['SalePrice'], ax=ax3) #'/2' for graph scaling
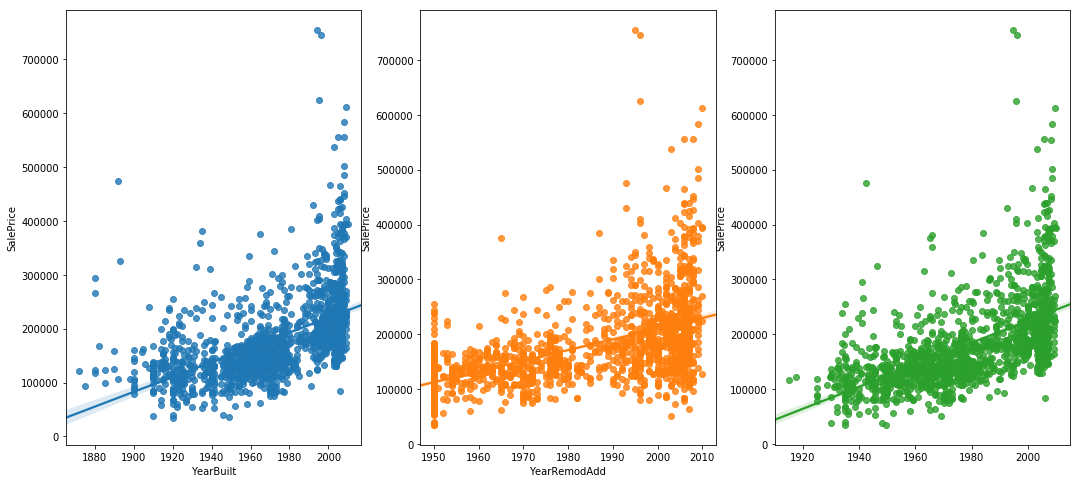
건축 연도와 리모델링 연도의 평균을 구하는것이 무슨 의미가 있나 할 수도 있다. 하지만 아주 의미가 없지는 않다! 건축 연도가 오래 되었어도, 최근에 리모델링을 하면 이 값이 높게 나왔을 것이고, 건축 이후 리모델링을 하지 않았다면 이 값은 아주 낮게 나왔을 것이다. 따라서 이 값이 높은 집들은 '지어진지 얼마 되지 않은 신축 건물 + 최근에 리모델링까지함' 에 가깝고, 이 값이 낮은 집들은 '오래된 건물 + 리모델링도 안함' 에 가까울 것이다. 그리고 이는 집값에 유의미한 영향이 있다고 초록색 그래프를 보면 판단할 수 있다.
all_data['YrBltAndRemod']=all_data['YearBuilt']+all_data['YearRemodAdd']
이를 'YrBltAndRemod' 라는 이름으로 두 개의 변수를 저장하자.
2.5. 자료형 수정 (Correcting Dtypes)
all_data['MSSubClass']=all_data['MSSubClass'].astype(str)
all_data['MoSold']=all_data['MoSold'].astype(str)
all_data['YrSold']=all_data['YrSold'].astype(str)
데이터 설명을 보면 나와있지만 'MSSubClass' 는 사실 숫자로 이루어진 데이터지만, 각각의 숫자가 의미를 갖고있는 범주형 변수이다. 그리고 판매 연, 월도 역시 연산 개념을 적용하는데는 무리가 있다. 그래서 이들을 문자열로 바꿔주자.
나는 사람들이 집을 볼때, 1층을 쭉 둘러보고 '괜찮네', 2층을 보고 '별로네', 지하층과 차고를 보고 '좋네' 이런식으로 판단할 거라고 생각했다. 이 평가의 기준 점수는 사람마다 약간 다르겠지만, 해당 시설물을 종합적으로 보고 판단하는 행위는 모두가 비슷할 것이라 생각했다. 나는 그래서 집의 시설물들을 묶어서 점수를 매기기로 했다.
2.6. 지하실 점수 (Bsmt)
Basement = ['BsmtCond', 'BsmtExposure', 'BsmtFinSF1', 'BsmtFinSF2', 'BsmtFinType1', 'BsmtFinType2', 'BsmtQual', 'BsmtUnfSF', 'TotalBsmtSF']
Bsmt=all_data[Basement]
지하실에 관한 변수들을 묶어서 저장하고,
Bsmt=Bsmt.replace(to_replace='Po', value=1)
Bsmt=Bsmt.replace(to_replace='Fa', value=2)
Bsmt=Bsmt.replace(to_replace='TA', value=3)
Bsmt=Bsmt.replace(to_replace='Gd', value=4)
Bsmt=Bsmt.replace(to_replace='Ex', value=5)
Bsmt=Bsmt.replace(to_replace='None', value=0)
Bsmt=Bsmt.replace(to_replace='No', value=1)
Bsmt=Bsmt.replace(to_replace='Mn', value=2)
Bsmt=Bsmt.replace(to_replace='Av', value=3)
Bsmt=Bsmt.replace(to_replace='Gd', value=4)
Bsmt=Bsmt.replace(to_replace='Unf', value=1)
Bsmt=Bsmt.replace(to_replace='LwQ', value=2)
Bsmt=Bsmt.replace(to_replace='Rec', value=3)
Bsmt=Bsmt.replace(to_replace='BLQ', value=4)
Bsmt=Bsmt.replace(to_replace='ALQ', value=5)
Bsmt=Bsmt.replace(to_replace='GLQ', value=6)
이들을 인코딩해준다. 이 'Po', 'Fa', ... 등의 값은 data description 텍스트 파일에 나와있다. 따라서 순서대로 좋은 값에는 높은 숫자를, 안좋은 값에는 낮은 숫자를, 지하실이 없으면 0을 입력해주었다.
Bsmt['BsmtScore']= Bsmt['BsmtQual'] * Bsmt['BsmtCond'] * Bsmt['TotalBsmtSF']
all_data['BsmtScore']=Bsmt['BsmtScore']
Bsmt['BsmtFin'] = (Bsmt['BsmtFinSF1'] * Bsmt['BsmtFinType1']) + (Bsmt['BsmtFinSF2'] * Bsmt['BsmtFinType2'])
all_data['BsmtFinScore']=Bsmt['BsmtFin']
all_data['BsmtDNF']=(all_data['BsmtFinScore']==0)
그리고 몇개 항목들을 곱해서 'BsmtScore' 항목을 만들어 지하실의 전반적인 상태를 복합적으로 평가할 수 있는 변수를 만들었다. 'BsmtFin' 변수는 지하실이 공사중이라면, 완성 면적과 상태를 포함하는 변수이다.
'BsmtFinScore' 은 지하실의 완성도 점수, 'BsmtScore' 은 지하실의 종합 점수, 'BsmtDNF' 는 지하실의 미완성 여부를 나타내는 변수이다.
2.7. 토지 점수 (Lot)
lot=['LotFrontage', 'LotArea','LotConfig','LotShape']
Lot=all_data[lot]
Lot['LotScore'] = np.log((Lot['LotFrontage'] * Lot['LotArea'])+1)
all_data['LotScore']=Lot['LotScore']
비슷한 상태도 토지면적과 모양, 접근성 등등을 고려할 수 있는 점수를 만들어 'LotScore' 로 저장하고
2.8. 차고 점수 (Garage)
garage=['GarageArea','GarageCars','GarageCond','GarageFinish','GarageQual','GarageType','GarageYrBlt']
Garage=all_data[garage]
all_data['NoGarage']=(all_data['GarageArea']==0)
차고에 관해서도 같은 방법으로 실행했다.
Garage=Garage.replace(to_replace='Po', value=1)
Garage=Garage.replace(to_replace='Fa', value=2)
Garage=Garage.replace(to_replace='TA', value=3)
Garage=Garage.replace(to_replace='Gd', value=4)
Garage=Garage.replace(to_replace='Ex', value=5)
Garage=Garage.replace(to_replace='None', value=0)
Garage=Garage.replace(to_replace='Unf', value=1)
Garage=Garage.replace(to_replace='RFn', value=2)
Garage=Garage.replace(to_replace='Fin', value=3)
Garage=Garage.replace(to_replace='CarPort', value=1)
Garage=Garage.replace(to_replace='Basment', value=4)
Garage=Garage.replace(to_replace='Detchd', value=2)
Garage=Garage.replace(to_replace='2Types', value=3)
Garage=Garage.replace(to_replace='Basement', value=5)
Garage=Garage.replace(to_replace='Attchd', value=6)
Garage=Garage.replace(to_replace='BuiltIn', value=7)
Garage['GarageScore']=(Garage['GarageArea']) * (Garage['GarageCars']) * (Garage['GarageFinish']) * (Garage['GarageQual']) * (Garage['GarageType'])
all_data['GarageScore']=Garage['GarageScore']
'GarageScore' 변수로 차고의 종합 점수를 판단할 수 있도록 했다.
2.9. 기타 변수 (Other Features)
1) 비정상적으로 하나의 값만 많은 변수들 삭제
all_data=all_data.drop(columns=['Street','Utilities','Condition2','RoofMatl','Heating'])
2) 비정상적으로 빈 값이 많은 변수들 삭제
집에 수영장 있는 집이 몇집이나 되겠는가...
그래프를 띄워보면 참 어이가 없다.
figure, (ax1, ax2) = plt.subplots(nrows=1, ncols=2)
figure.set_size_inches(14,6)
sns.regplot(data=data, x='PoolArea', y='SalePrice', ax=ax1)
sns.barplot(data=data, x='PoolQC', y='SalePrice', ax=ax2)
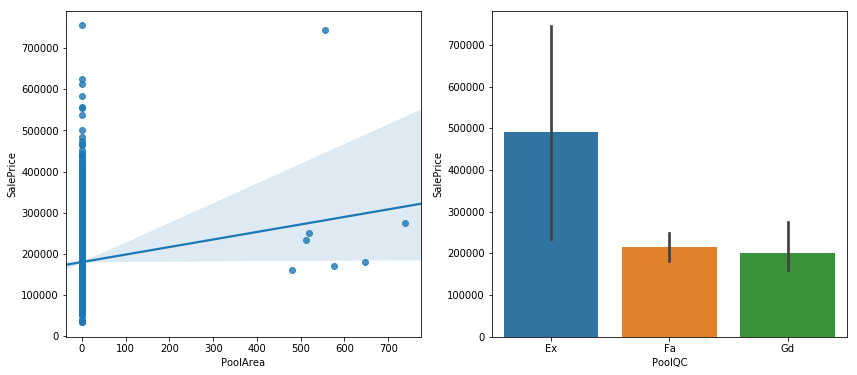
all_data=all_data.drop(columns=['PoolArea','PoolQC'])
.
figure, (ax1, ax2) = plt.subplots(nrows=1, ncols=2)
figure.set_size_inches(14,6)
sns.regplot(data=data, x='MiscVal', y='SalePrice', ax=ax1)
sns.barplot(data=data, x='MiscFeature', y='SalePrice', ax=ax2)
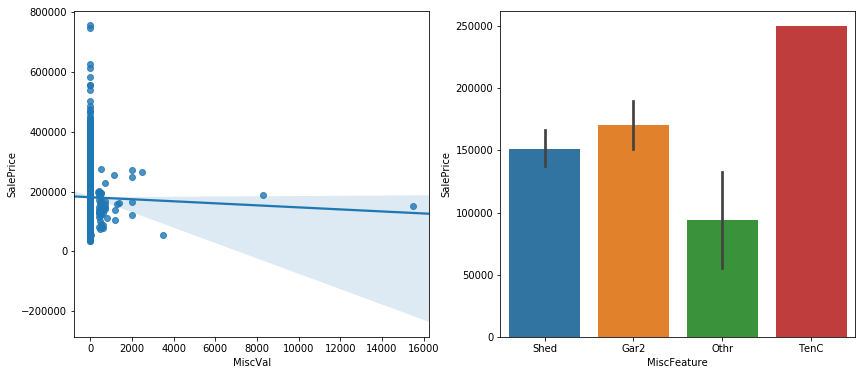
all_data=all_data.drop(columns=['MiscVal','MiscFeature'])
3) 위 둘보다는 낫지만, (채워진)결측치가 많은 경우
sns.regplot(data=data, x='LowQualFinSF', y='SalePrice')
sns.regplot(data=data, x='OpenPorchSF', y='SalePrice')
sns.regplot(data=data, x='WoodDeckSF', y='SalePrice')
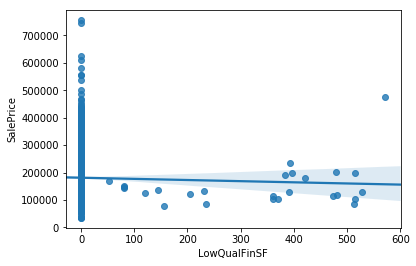
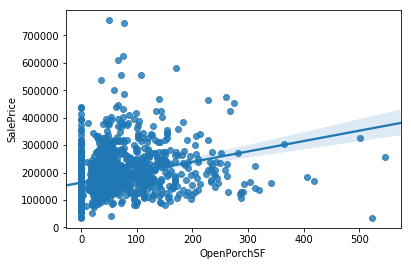
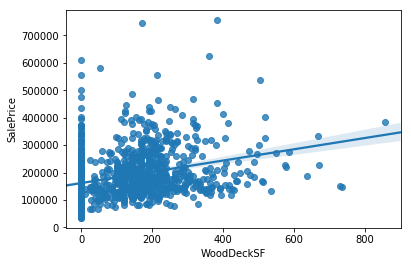
이들은 0 값만 분리해주면 결과가 나쁘지 않을 것 같아서 0만 분리해주었다.
all_data['NoLowQual']=(all_data['LowQualFinSF']==0)
all_data['NoOpenPorch']=(all_data['OpenPorchSF']==0)
all_data['NoWoodDeck']=(all_data['WoodDeckSF']==0)
3. 전처리 (Preprocessing)
3.1. 범주형 변수 (Categorical Feature)
범주형 변수가 상당히 많은 데이터셋이다. 범주형 변수를 다짜고짜 1, 2, 3, 4, ... 로 인코딩해주면 머신러닝이 오해할 소지가 충분하기 때문에 일단 모조리 원핫 인코딩을 한다.
non_numeric=all_data.select_dtypes(np.object)
def onehot(col_list):
global all_data
while len(col_list) !=0:
col=col_list.pop(0)
data_encoded=pd.get_dummies(all_data[col], prefix=col)
all_data=pd.merge(all_data, data_encoded, on='Id')
all_data=all_data.drop(columns=col)
print(all_data.shape)
onehot(list(non_numeric))
3.2. 수치형 변수 (Numeric Feature)
수치형 변수는 비대칭이 너무 심해지지 않게끔, Right Skewed 가 크게 되어있는 데이터들에만 로그를 씌워 적절히 변형시켜준다.
numeric=all_data.select_dtypes(np.number)
def log_transform(col_list):
transformed_col=[]
while len(col_list)!=0:
col=col_list.pop(0)
if all_data[col].skew() > 0.5:
all_data[col]=np.log(all_data[col]+1)
transformed_col.append(col)
else:
pass
print(f"{len(transformed_col)} features had been tranformed")
print(all_data.shape)
log_transform(list(numeric))
그럼 이제 데이터를 나누는 일만 남았다. all_data 항목을 test 데이터의 갯수에 맞게끔 잘라서 따로 저장하면 된다.
print(train.shape, test.shape)
Xtrain=all_data[:len(train)]
Xtest=all_data[len(train):]
print(Xtrain.shape, Xtest.shape)
(1458, 79) (1459, 79)
(1458, 309) (1459, 309)
원래 train 데이터의 갯수와 데이터를 가공한 Xtrain 데이터의 갯수가 1458 개로 동일하다. (위에서 'GrLivArea' 할 때 train 에서 두개를 지웠었다!)
4. 머신러닝 모델로 학습
from sklearn.linear_model import ElasticNet, Lasso
from sklearn.ensemble import GradientBoostingRegressor
from sklearn.pipeline import make_pipeline
from sklearn.preprocessing import RobustScaler
from xgboost import XGBRegressor
import time
import optuna
from sklearn.model_selection import cross_val_score
from sklearn.metrics import mean_squared_error
model_Lasso= make_pipeline(RobustScaler(), Lasso(alpha =0.000327, random_state=18))
model_ENet = make_pipeline(RobustScaler(), ElasticNet(alpha=0.00052, l1_ratio=0.70654, random_state=18))
model_GBoost = GradientBoostingRegressor(n_estimators=3000, learning_rate=0.05, max_depth=4, max_features='sqrt', min_samples_leaf=15,
min_samples_split=10, loss='huber', random_state=18)
model_XGB=XGBRegressor(colsample_bylevel=0.9229733609038979,colsample_bynode=0.21481791874780318,colsample_bytree=0.607964318297635,
gamma=0.8989889254961725, learning_rate=0.009192310189734834, max_depth=3, n_estimators=3602,
reg_alpha=3.185674564163364e-12,reg_lambda=4.95553539265423e-13, seed=18, subsample=0.8381904293270576,
tree_method='gpu_hist',verbosity=0)
Lasso, ElasticNet, sklearn의 GradientBoosting, XGBoost 4개의 모델을 불러와서 저장한다. 최종 예측 결과물은 이 4개의 모델의 예측값의 평균값을 사용해 제출할 것이다.
model_Lasso.fit(Xtrain, Ytrain)
Lasso_predictions=model_Lasso.predict(Xtest)
train_Lasso=model_Lasso.predict(Xtrain)
model_ENet.fit(Xtrain, Ytrain)
ENet_predictions=model_ENet.predict(Xtest)
train_ENet=model_ENet.predict(Xtrain)
model_XGB.fit(Xtrain, Ytrain)
XGB_predictions=model_XGB.predict(Xtest)
train_XGB=model_XGB.predict(Xtrain)
model_GBoost.fit(Xtrain, Ytrain)
GBoost_predictions=model_GBoost.predict(Xtest)
train_GBoost=model_GBoost.predict(Xtrain)
log_train_predictions = (train_Lasso + train_ENet + train_XGB + train_GBoost)/4
train_score=np.sqrt(mean_squared_error(Ytrain, log_train_predictions))
print(f"Scoring with train data : {train_score}")
log_predictions=(Lasso_predictions + ENet_predictions + XGB_predictions + GBoost_predictions) / 4
predictions=np.exp(log_predictions)-1
submission['SalePrice']=predictions
submission.to_csv('Result.csv')
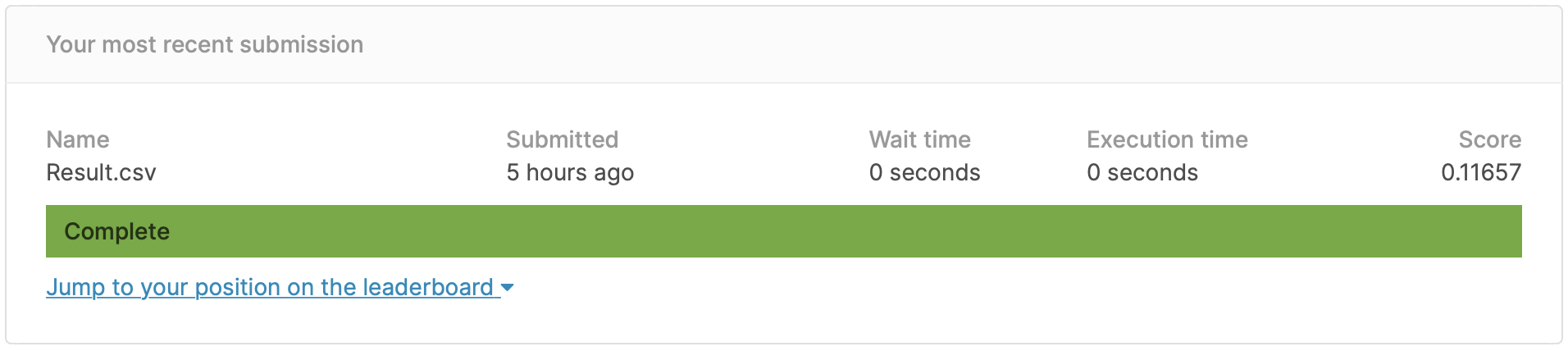
0.11657 정도의 점수가 나올 것이다. 대략 4600명 중에서 약 517등. 상위 약 12퍼센트 정도의 점수이다. (2020년 3월 4일 기준)